Unlocking Potential: The Role of Medical Datasets in Machine Learning
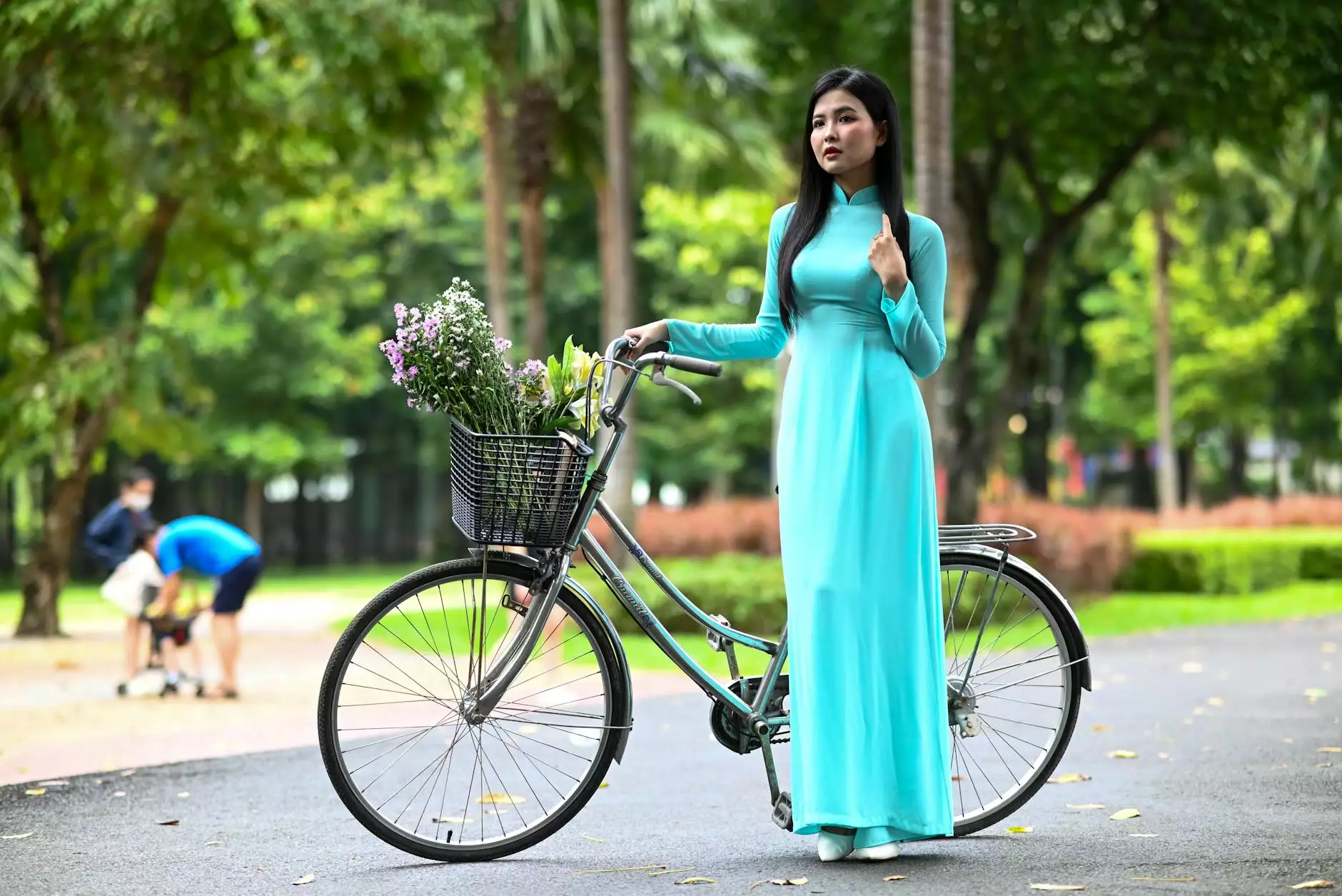
In today's rapidly evolving technological landscape, the intersection between medicine and artificial intelligence has never been more prominent. A key driver of this intersection is the availability of medical datasets for machine learning, which hold vast potential for revolutionizing healthcare systems globally. This article will delve deep into the significance of these datasets, their types, and their applications in improving patient outcomes.
The Importance of Medical Datasets for Machine Learning
As the world generates a staggering amount of medical data, the ability to harness this information through machine learning becomes crucial. The integration of medical datasets into machine learning algorithms can lead to breakthroughs that enhance diagnostics, personalize treatments, and ultimately improve patient care.
What are Medical Datasets?
Medical datasets refer to structured collections of health-related data, which may include anything from patient demographics to clinical outcomes. These datasets can be compiled from various sources, including:
- Clinical Trials: Organizing patient data during studies aimed at testing new treatments.
- Electronic Health Records (EHRs): Comprehensive records provided by healthcare facilities for each patient.
- Genomic Databases: Detailed information about genetic variations and their implications in health.
- Public Health Data: Statistics and data on health outcomes provided by health organizations and agencies.
Types of Medical Datasets for Machine Learning
Understanding the various types of medical datasets is essential for leveraging them effectively in machine learning applications. Here are some prominent types:
1. Patient Health Records
These records provide a wealth of information about patient visits, diagnoses, treatments, and outcomes. By analyzing large volumes of patient data, machine learning models can identify patterns that may assist in predicting diseases or determining the effectiveness of treatment methods.
2. Imaging Data
Data collected from radiological studies, such as MRIs, CT scans, and X-rays, are invaluable. Advances in computer vision allow machine learning models to interpret imaging data with remarkable accuracy. This can lead to earlier detection of conditions such as cancer, where every moment counts.
3. Genomic Sequencing Data
With the completion of initiatives like the Human Genome Project, vast genomic datasets have become available. Machine learning can analyze these datasets to find correlations between genetic factors and disease susceptibility or treatment response, paving the way for personalized medicine.
4. Clinical Trial Data
Clinical sessions generate extensive datasets each time a new drug is tested. Machine learning can analyze this data to identify trends that may inform future clinical practice and help in determining which treatments work best for specific populations.
Applications of Medical Datasets in Machine Learning
The applications of medical datasets for machine learning are extensive, transforming various aspects of healthcare. Here are a few key applications:
1. Disease Prediction and Diagnosis
Machine learning algorithms trained on comprehensive datasets can analyze symptoms and predict diseases before they manifest clinically. This can be particularly beneficial for chronic diseases such as diabetes and heart disease, where early detection can significantly impact treatment success.
2. Personalized Treatment Plans
By examining patient data, machine learning can help create tailored treatment regimens that cater to individual responses to therapies. This not only enhances the likelihood of positive outcomes but also helps in minimizing side effects.
3. Drug Discovery and Development
Medical datasets provide insights that accelerate drug discovery. Machine learning models can predict how potential drug compounds interact at the molecular level, which significantly cuts down on the time and cost associated with bringing new drugs to market.
4. Predictive Analytics for Patient Care
With access to historical patient data, machine learning can forecast various future health outcomes. This predictive capability is essential for managing healthcare resources and ensuring that patients receive timely interventions.
The Challenges of Using Medical Datasets
While the use of medical datasets for machine learning presents numerous opportunities, several challenges must be addressed:
1. Data Privacy and Security
Medical data involves sensitive personal information, and protecting this data is paramount. Compliance with regulations such as HIPAA in the U.S. is essential to ensure patient confidentiality while using their data for research and machine learning.
2. Data Quality and Standardization
The accuracy and consistency of medical datasets can vary significantly. Ensuring high-quality data is crucial for training effective machine learning models. Standardizing data collection and management practices across institutions can alleviate some of these issues.
3. Interpretability of Machine Learning Models
Many machine learning models, especially deep learning algorithms, operate as "black boxes." Healthcare practitioners need to understand how models make predictions to trust their guidance, leading to increased demand for explainable AI solutions in medicine.
The Future of Medical Datasets in Machine Learning
As technology continues to evolve, the potential of medical datasets for machine learning will only expand. With the advent of novel approaches such as federated learning, which allows machine learning models to learn from decentralized data without compromising privacy, the possibilities are endless.
Emphasizing Collaborative Efforts
Collaboration between healthcare providers, researchers, and technology developers will be crucial to maximize the potential of medical datasets. Sharing knowledge and resources can lead to significant advancements in AI-driven healthcare innovations.
Integrating AI into Routine Healthcare
As the healthcare industry increasingly adopts AI technologies, integrating machine learning models into routine practices will aid in enhancing diagnostic capabilities and streamlining treatment approaches. This integration promises to create more overwhelmed healthcare systems by improving efficacy and opening pathways for preventative care.
Conclusion
The landscape of healthcare is evolving rapidly, and the role of medical datasets in machine learning is pivotal in driving this transformation. By harnessing these datasets, the medical community stands on the brink of groundbreaking advancements that can change the way diseases are predicted, diagnosed, and treated.
The fusion of data science and medicine through machine learning can usher in an era of personalized health solutions, radically improving patient outcomes and creating a more efficient healthcare system. As we continue to unlock the potential of medical datasets for machine learning, the future looks bright, promising better health for all.
medical dataset for machine learning